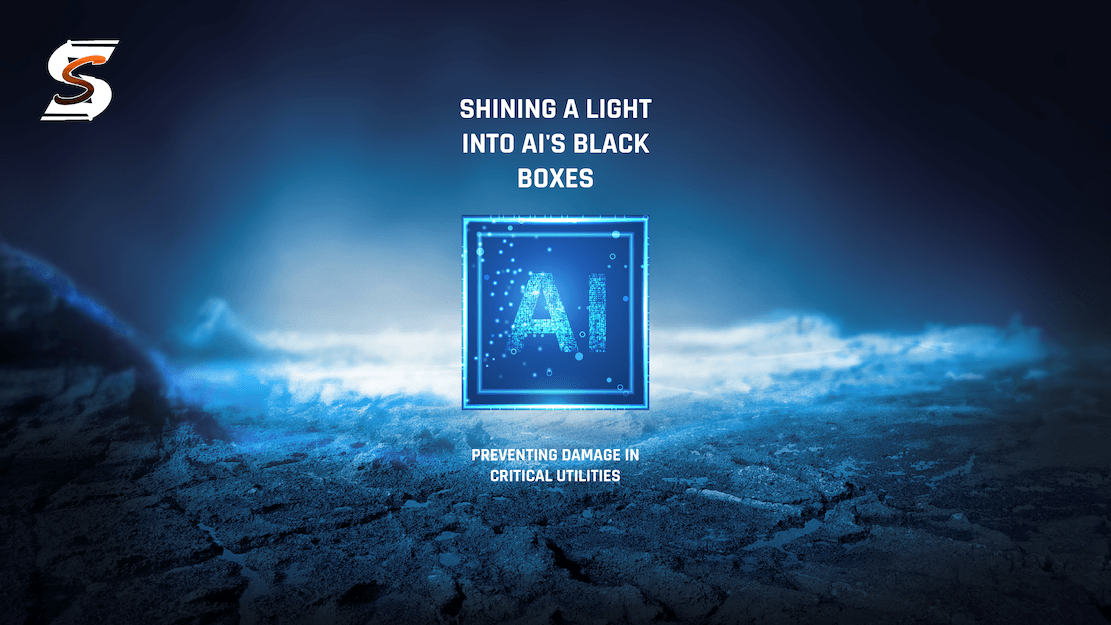
As artificial intelligence systems are increasingly deployed in the utility and damage prevention sectors, a major challenge looms: the opacity and lack of transparency in many AI models used for these tasks. The "black box" nature of complex machine learning algorithms like deep neural networks makes it extremely difficult to understand the reasoning and decision-making process behind their outputs. This poses a serious risk when the AI system's decisions could lead to service disruptions, equipment damage, or even public safety hazards. In order to build trust with the technology we need users to understand how it is making decisions, what the limits are and what the inputs are.
Take for example an "AI agent" trained to dynamically control locate dispatching across a geographic area using risk scoring as it's main decision making algorithm. There's lots to gain with AI and by pinpointing the highest risk areas in advance, utilities can intelligently prioritize their damage prevention resources and processes for maximum safety impact. As the AI models become more accurate by training on more data over time, human workers could be guided towards essentially zero-strike work by only allowing digging to proceed in predicted low-risk areas. For utilities, this could translate into millions saved annually by preventing costly strikes, service disruptions, and safety incidents. Applying smart AI modelling for predictive risk scoring represents a huge step forward in excavation damage prevention. While this AI may exceed human capabilities at this task, a lack of insight into its decision logic creates a frightening blind spot. What if there are edge cases or anomalies that cause the system to make disastrously incorrect judgments, potentially triggering widespread outages or overloads? Without interpretability into how the AI arrives at its outputs, it's tremendously difficult to validate safe and reliable operation.
The consequences of AI opacity extend across many other vital services like water distribution, traffic control systems, chemical processing plants, and more. A single erroneous decision from an inscrutable AI system could have catastrophic downstream effects. Exposed to adversarial attacks or subtle distribution shifts, these "black boxes" may react in ways that humans cannot anticipate or safeguard against.
Understandably, the notion of entrusting critical infrastructure to fundamentally opaque systems with limited safety controls is a deeply concerning proposition for utilities providers, regulators, and the public. While the efficiency and optimization benefits of AI are powerful, they could be outweighed by the risks of a "black box" causing damage or disruption that could impact millions. We need to build trust...
So how can we strengthen transparency and validation capabilities to harness the power of AI in the utility industry while preventing worst-case damage scenarios? A few key paths forward:
-
Interpretable Model Design: Rather than defaulting to opaque neural networks, utilities infrastructure should prioritize inherently interpretable AI models based on more transparent architectures like linear models, decision trees, or rule induction. While these sacrifice some performance, the transparency enables rigorous testing.
-
Human-AI Hybridization: Critical high-stakes decisions should not be fully autonomized, but rather operate with a "human-in-the-loop" validation component before execution. AI serves as a powerful aide but doesn't have ultimate authority.
-
Saliency Mapping: Advanced visualization techniques can help identify what input factors were most influential in an AI's decision-making process, providing some window into its logic.
-
Sandbox & Simulation Testing: AI systems for damage prevention should undergo extensive testing in sandboxed environments and simulations across all possible scenarios before any real-world deployment.
While vital, these interpretability and validation methods are limited. Fundamentally reorienting AI development incentives toward simpler, modular, and inherently interpretable model architectures from the ground up - rather than inscrutable, monolithic models - may be necessary for the highest stakes applications.
As AI's role in damage prevention for critical systems grows, meaningful windows into these systems' reasoning and decisions cannot be an afterthought. Transparent and interpretable AI may sacrifice some theoretical capabilities, but represents a worthy tradeoff to ensure public safety, reliability and trust as these transformative technologies are integrated into utilities that billions depend upon. Removing the opacity of AI black boxes must be a key priority to realize AI's damage prevention potential responsibly.
Share this Post